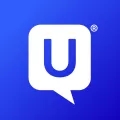
We spend a lot of our time analyzing the data, identifying insights, and making recommendations.
As part of this process, we often talk to our clients about the statistics they can run on the data collected in UserTesting and what insights they can infer. Some of the most common questions we get asked are: What does statistical significance mean? What is a p-value? When should we draw conclusions from the differences in the data? And when shouldn’t we?
In this post we'll talk about what a p-value actually is, why it has to be less than 0.05, and how this can help with making decisions in your UX research.
A great way of understanding your data is to run an appropriate statistical test. Let’s take a scenario where you want to know which of two new prototypes is easier to use.
You could set up a research study where participants complete a task on each prototype for one of your key user journeys. Then you'd ask participants to rate the prototypes' usability after they complete a task.
As a UserTesting client, you're able to then look at the study results and see that Prototype A has an average ease-of-use rating of 5.6 out of 7, while Prototype B gets an average (mean) of 5.9 out of 7.
What can you say about this finding?
In our example, we assume a rating of 1 reflects hard to use, and 7 reflects easy to use. Prototype B therefore has the higher mean, so participants rated it, on average, easier to use.
But does a higher mean rating for Prototype B actually represent anything? Is 5.9 so much greater than the 5.6 that we can confidently say Prototype B is easier to use? How big does a difference need to be? Here, the difference between the means (the mean difference) is 0.3. Are we prepared to dismiss Prototype A just because on average it was rated 0.3 lower than Prototype B?
Rather than just relying on your data to only show you descriptive information (e.g. means, percentages etc.), running statistical tests can help you to make confident decisions about the content you're testing. In this scenario, is one of the prototypes going to be easier to use than the other?
There are many different statistical tests out there, and the one you use depends on the design of your study and the type of data you have collected. One thing they have in common is the p-value. The p-value helps us to decide if we have strong enough evidence to make conclusions about our data. In the context of our difficulty ratings, we would be testing whether the difference in our means (0.3) is large enough to suggest there is something distinct about our prototypes — that there's something about Prototype B that makes it easier to use than Prototype A.
The p-value we obtain from running a statistical test on this data is the probability of obtaining this mean difference if we assume that there is no difference between our prototypes to find. In the case of our ease of use means, the p-value tells us the probability of obtaining the difference between our means if there truly was no difference between the prototypes. As with other statistical tests, what we require is enough evidence to be happy to conclude that our mean difference is there.
If we obtain a p-value of less than 0.05 from our statistical test, we're happy to conclude there is a difference between our means, and that they're statistically significantly different. 0.05 is the cut-off set by convention.
So what does p < 0.05 mean and why does p have to be less than 0.05?
By setting the p-value at 0.05, we are saying there is only a 5% chance, or less, of obtaining the result from our statistical test, when there isn’t actually a difference there. If the p-value we obtain is less than 0.05, there is less than a 5% chance that the difference we have found isn’t really there and we have only found this difference by mistake.
In contrast, if our statistical test came back with a p-value of 0.08, as this is greater than 0.05, and we would conclude that there is no statistically significant difference between our means.
If we set the p-value to be 0.01, then we would only be happy to accept a finding that could only occur 1% of the time, or less, if the difference wasn’t really there. Where we set the p-value determines how strict we want to be about saying there is a mean difference when there actually isn’t one there. The p-value doesn’t have to be 0.05 but typically in statistics it is the cut-off we are happy to live with.
In our comparison between two prototypes, a statistically significant difference between Prototype A and Prototype B allows us to recommend Prototype B as the easier-to-use design with a higher level of confidence. Our next steps could be to test this prototype vs the current site, to provide confidence that implementing this new design would help your customers with this key journey.
If the difference between the two prototypes was not statistically significant, we could not say that either prototype was easier to use. The range of options open to us then would be to look at other UX metrics we have collected: do the Success rates tell us a different story? Does the behavioral data of the participants screens highlight any difficulties they encountered? Were these difficulties similar on both prototypes? What do the participants’ open verbatim comments tell us?
Not finding a statistically significant result is not the end of the world! Insights we can gather from the wealth of behavioral and attitudinal metrics help us discover which elements to take from the current prototypes, in order to iterate the design. Taking the positives and removing any usability issues from Prototypes A and B, we’d be ready to test these new iterations in future studies.
So next time you want to confidently say to your design team, your developers or your Manager, that we should go with this prototype or this design, and you need to give them a reason why, remember: the p-value is there to help.